Advanced Bayesian Methods
Presenter(s): Dr Gabriel Katz
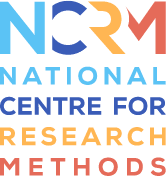
This resource looks at modern Bayesian computation. Focusing on the two most widely used Bayesian algorithms, the Gibbs Sampler, and the Metropolis-Hastings. It reviews s criteria used to assess model convergence, and when running Bayesian models, how to identify that the model is ready to be used to draw inferences about parameters. It discusses the goodness of fit criteria used in the Bayesian world, which differs from those used in frequency statistics. It concludes by discussing methods to speed up conversion or speed up execution time. All videos in this resource uses the a single slide set, which can be accessed here.
Introduction
In this video, Gabriel Katz, Associate Professor of Politics and Quantitative Methods at the University of Exeter introduces this online resource which will explore fundamental aspects of modern Bayesian computation.
The Basics of Bayesian Computation
In this video, Gabriel goes over the basics of Bayesian computation, working through a series of exercises.
Metropolis-Hastings
In this video, Gabriel looks at the second main algorithm used in Bayesian computations, which is the Metropolis Hastings algorithm and can be used when sampling from the conditional distributions is not possible.
Gibbs Sampling
In this video, Gabriel illustrates the Gibbs Sampling algorithm and how it can be used to break down a complicated problem into a series of issue conditional problems.
Speeding up Bayesian computations
In this video, Gabriel talks though how researchers are able to use Bayesian methods to speed up calculations and reduce the time taken, whether that is using c+++, cluster computing services or cloud computing.
Assessing Convergence
In this video, Gabriel explores the issue of convergence and how we assess convergence when estimating models in a Bayesian fashion using modern Bayesian computational approaches. This includes using traceplots and chains starting from different initial values.
About the author
Gabriel earned his PhD from the California Institute of Technology (Caltech) in June 2010 and aslo holds an MSc. from Caltech and a Licenciatura in Economics from the Universidad de la Republica, in Uruguay. Gabriel's work focuses on Latin American Politics, Political Behavior, Political Economy and Research Methods, and has been published in leading economics and political science journals such as the American Journal of Political Science, Comparative Political Studies, the Economic Journal and the Journal of Law, Economics & Organization, among others. Gabriel's research uses advanced quantitative methods - mainly Bayesian statistics, but also causal inference and experimental techniques - to address substantive questions in comparative politics, political behaviour and political economy.
- Published on: 1 July 2021
- Event hosted by: University of Exeter
- Keywords: Quantitative Data Handling and Data Analysis | Statistical Theory and Methods of Inference | Bayesian methods |
- To cite this resource:
Dr Gabriel Katz. (2021). Advanced Bayesian Methods. National Centre for Research Methods online learning resource. Available at https://www.ncrm.ac.uk/resources/online/all/?main&id=20777 [accessed: 4 July 2025]
⌃BACK TO TOP