Mixture and Group-Based Trajectory Models
Presenter(s): Oliver Perra
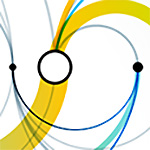
This resource illustrates key concepts and processes of mixture and trajectory-based group models, specifically Growth Mixture Models (GMM) and Latent Class Growth Analysis (LCGA), with examples from research and exercises using Mplus software. Solutions to the exercises are also provided.
GMM and LCGA are Mixture Models applied in order to identify a limited but adequate number of participant categories that follow distinctive developmental trajectories in one or more processes. These methods are based on Latent Growth Curve models. Latent Growth Curve models describe change in observed variables (continuous, categorical, or count variables) as a function of latent growth factors: these factors thus represent continuous change in the outcome as a function of time. GMM and LCGA extend the latent growth curve models by assuming that the population is made up of a mix of individuals that display distinctive trajectories. These sub-populations share the same propensity to display a specific developmental trajectory, but the trajectory of individuals within a sub-population differ substantially from the trajectories of individuals in other sub-populations. GMM and LCGA therefore marry a person-centred approach with a variable-centred approach: As well as measuring the change in observed variables, GMM LCGA also identify groups of people that behave in similar ways. These approaches are particularly useful in disciplines that are interested in identifying sub-populations that differ in their developmental trajectories. For example, many authors that study mental health development assume that are marked individual differences whereby some individuals present chronical mental health problems but others never present them, or present them only in particular periods or circumstances. This heterogeneity may also reflect qualitative differences between individuals, often associated with differences in causal mechanisms, differences in other processes, differences in later attainments. GMM and LCGA provide formal methods to identify these different subpopulations and investigate their associations with predictors, other processes, and distal outcomes.
The purpose of GMM and LCGA
The mixture model approach underlying GMM and LCGA contrasts with the assumption of latent growth curve models. While latent growth curve models assume that all individuals in a study follow the same trajectory of development in an outcome, GMM and LCGA assumes that the study population is made up of a finite number of groups, each one of these groups with distinctive developmental trajectories. These trajectory-based groups are latent classes, that is, distinctive “types” of individuals that within each class share the a propensity for following a specific trajectory. These classes of individuals are not directly observed, but they can be identified following probability methods. Latent classes are categorical latent variables that explain differences in individuals’ trajectories. Latent classes are exhaustive: every individual will be in one trajectory group or another. Another assumption is that each person belongs to one and only one of the latent classes: classes are mutually exclusive. Since GMM and LCGA are based on latent class probability methods, allocation of individuals into trajectory groups is estimated with uncertainty. Different models will provide more or less uncertain classifications, and the estimation of how precise is the classification afforded by a model is one of the advantages of these methods.
The key difference between GMM and LCGA lies in their assumptions about inter-individual variability within the classes. GMM assumes that there is some variability within the latent classes: within trajectory groups, individuals still show some variability around the class-specific average trajectory. LCGA instead assumes that there is no variability in the trajectories within classes and individuals follow the same class-specific pattern.
GMM and LCGA applied to categorical variables
When investigating developmental trajectories researchers often deal with ordered categorical variables where categories of responses are not evenly spaced and distributions are asymmetric. Latent Growth Curve models have been developed that can successfully deal with this type of variables using a Logit-Link and a Latent Response formulation. Expanding these approaches to the case of Mixture models allows the application of GMM and LCGA models to categorical and dichotomous variables.
GMM and LCGA with covariates and distal outcomes, and further applications
GMM and LCGA models offer possibilities to include both time-invariant and time-varying covariates in the models. Covariates can be included as predictors of latent class affiliation by modelling the multinomial logistic regression of latent classes on covariates. These methods also allow investigating the changes in latent class probabilities associated with different combinations of covariates values, thus allowing investigation of the cumulative effects of exposure to risk factors. Distal outcomes can be introduced in the models. The latter task has been facilitated by the devising of an approach that separates the latent class model estimation from the investigation of structural relationships between latent classes and covariates or distal outcomes, the Three-Step method. GMM and LCGA also offer flexible approaches to investigate associations between individual differences in the developmental trajectories of multiple processes (e.g. mental health and drug use) as well as methods to identify individuals that differ in trajectories across multiple variables.
___
Download the exercise files (Zip folder).
Exercises with solutions, datasets, and information about: Mixture and Trajectory-Based Groups using Mplus.
About the author
Dr Oliver Perra is a lecturer at the School of Nursing and Midwifery, Queen’s University Belfast. His research revolves around the early experiences that explain differences in children’s adaptation and socio-cognitive abilities. He explores these issues by applying a transactional approach: this allows to investigate how interactions between children's characteristics and modifiable environmental factors can affect children's developmental pathways.
- Published on: 20 November 2023
- Event hosted by: Queen's University Belfast
- Keywords: Mixture | Group-Based | Trajectory Models | Growth Mixture Models | Latent Class Growth Analysis | Mplus software | Latent Growth Curve models | person-centred approach | variable-centred approach | mental health development | ordered categorical variables | Logit-Link | covariates | distal outcomes | individual differences | multiple processes |
- To cite this resource:
Oliver Perra. (2023). Mixture and Group-Based Trajectory Models. National Centre for Research Methods online learning resource. Available at https://www.ncrm.ac.uk/resources/online/all/?id=20826 [accessed: 15 June 2025]
⌃BACK TO TOP