Introduction to Latent Class Analysis
Presenter(s): Oliver Perra
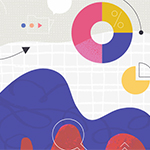
This resource shares key concepts and processes of Latent Class Analysis (LCA), with examples from research and exercises using Mplus software (solutions to the exercises are also provided).
LCA is a statistical method within the family of Mixture Models. Mixture models assume that a population is made up of sub-populations, a “mix” of individuals. These sub-populations share the same propensities to display some patterns of behaviour, and these propensities differ substantially from those of other sub-populations. The population observed is therefore heterogeneous, made up of different groups of people, however, this heterogeneity can be reduced to a limited number of types, groups, or classes. Sub-populations are not directly observed, but LCA provides probability-based methods to identify these sub-groups, using the individuals’ observed behaviours as indicators.
LCA is a person-centred approach: the focus is not on the relationships between variables, but rather on identifying groups or clusters of people that have similar characteristics or behave in similar ways. LCA is a measurement model because it assumes that what explains the variability we observe in people’s behaviour are latent variables. LCA is conceptually similar to other measurement models like factor analysis: the key difference between LCA and factor analysis is that the underlying variables explaining peoples’ behaviour patterns are categorical variables.
LCA provides methods that allow to:
- Decide on the number of sub-groups that explain the variability in observed behaviour patters;
- Describe and characterise these sub-groups;
- Assign persons to these different sub-groups, based on their observed pattern of behaviour.
Furthermore, LCA can be used to investigate covariates that can predict or influence the affiliation to sub-groups; LCA can also be used to investigate the associations between sub-groups and distal outcomes. For example, research has been used to identify patterns of mental health co-morbidity in adolescents, as well as the factors that influence affiliation to these co-morbidity sub-groups (e.g. gender, Socio-Economic Status, exposure to traumatic experiences). LCA can then be used to investigate, for example, if these co-morbidity sub-groups differ significantly in their response to a psychological intervention. Analyses of LCA with covariates and distal outcomes have become more accessible thanks to recent advancements in methods.
The purpose of LCA.
The main assumptions of LCA: persons in a sample belong to one latent class or another, i.e. latent classes are exhaustive and capture the whole of the sample.
Another assumption is that each person at a given time belongs to one and only one of the latent classes: classes are mutually exclusive.
Another assumption is that of intragroup homogeneity: individuals within a class share the same propensity to behaviour patterns, and these propensities differ from those of individuals in other classes. The main goals of LCA are to enumerate the latent classes, characterise them in terms of how these underlying classes influence observed behaviours, and categorise individuals in these classes, based on the observed behaviour patterns.
LCA allows to identify qualitative differences between individuals: latent classes can represent differences that go beyond differences along one dimension (e.g. Progressive/Conservative; Disordered/Non Disordered) but rather represent more nuanced, multi-dimensional differences.
Formal definition.
LCA is a probability model: the associations between latent classes and observed behaviour patterns are estimated with error, so these associations are probabilistic. Consequently, assignment of individuals into latent classes is also probabilistic and has a degree of uncertainty. The examples provided in the presentation will also illustrate how to test hypotheses about the model by imposing constraints on parameters.
Multiple Pseudo-Class Draws, and the Three-Step Approach.
The use of LCA with covariates and distal outcomes has been curtailed by the fact that the indicators-based measurement model shifts and changes when estimated together with auxiliary variables (covariates and distal outcomes). While a naïve solution to this problem has involved the use of individuals’ latent class affiliation as a variable in analysis, this solution is unsatisfactory because it fails to take into account the level of uncertainty in assigning individuals to latent classes, thus introducing bias in the analyses. More sophisticated and satisfactory solutions have been developed recently: the Multiple Pseudo-Class Draws, and the Three-Step Approach. The Multiple Pseudo-Class Draws uses methods akin to multiple imputation of missing values to create multiple draws of the most likely latent class affiliation, combining results across these draws using Rubin’s rule. The Three-Step Approach conducts the measurement model estimation in a first step, which is separate from the testing of associations between latent classes and auxiliary variables. In this way, the measurement model is not re-estimated when auxiliary variables are included. To control for uncertainty in latent class allocation, the latent class model in the analyses with auxiliary variables is fixed at measurement parameters that represent the level of uncertainty in the measurement model from the first step. The complementary material and the exercises will provide more information and examples of these methods.
___
Download the exercise files (Zip folder).
In the folder you will find an Introduction document to outline the available resources including an introduction to MPlus software, guidance on latent class models, practice data and exercises with solutions to the exercises.
___
Books and references:
Books
Two good books to get started with Latent Class Analysis (LCA) and go on to learn about its applications and uses are these:
Hagenaars, J., & McCutcheon, A. (Eds.). (2002). Applied Latent Class Analysis. Cambridge: Cambridge University Press. doi:10.1017/CBO9780511499531
Collins, L. M., & Lanza, S. T. (2009). Latent class and latent transition analysis: With applications in the social, behavioral, and health sciences. New York: John Wiley & Sons. doi:10.1002/9780470567333
McCutcheon also produced a very approachable introduction for SAGE:
McCutcheon, A. L. (1987). Latent class analysis. SAGE Publications. doi :10.4135/9781412984713
A recent book published for Springer:
Eshima, N. (2022). An Introduction to Latent Class Analysis. Singapore: Springer. https://doi.org/10.1007/978-981-19-0972-6
References concerning reporting of LCA results
Nylund-Gibson, K., & Choi, A. Y. (2018). Ten frequently asked questions about latent class analysis. Translational Issues in Psychological Science, 4(4), 440-461. https://doi.org/10.1037/tps0000176
Ryoo, J. H., Wang, C., Swearer, S. M., Hull, M., & Shi, D. (2018). Longitudinal model building using latent transition analysis: an example using school bullying data. Frontiers in psychology, 9, 675. https://doi.org/10.3389/fpsyg.2018.00675
Sinha, P., Calfee, C.S., & Delucchi, K.L. (2021). Practitioner's Guide to Latent Class Analysis: Methodological Considerations and Common Pitfalls. Critical Care Medicine, 49(1), e63-e79. doi: 10.1097/CCM.0000000000004710
References on approaches to include covariates and distal outcomes in LCA models
Asparouhov, T., & Muthén, B. (2014). Auxiliary variables in mixture modeling: Three-step approaches using Mplus. Structural Equation Modeling, 21, 329–341. doi:10.1080/10705511.2014.915181
Bray, B. C., Lanza, S. T., & Collins, L. M. (2010). Modeling relations among discrete developmental processes: A general approach to associative latent transition analysis. Structural Equation Modeling, 17, 541–569. doi:10.1080/10705511.2010.510043
Chung, H, Park, Y., & Lanza, S. T. (2005). Latent transition analysis with covariates: pubertal timing and substance use behaviours in adolescent females. Statistics in Medicine, 24, 2895–2910. doi:10.1002/sim.2148
Clark, S. L., & Muthén, B. (2009). Relating latent class analysis results to variables not included in the analysis. Retrieved from https://www.statmodel.com/download/relatinglca.pdf
Nylund-Gibson, K., Grimm, R., Quirk, M., & Furlong, M. (2014). A latent transition mixture model using the three-step specification. Structural Equation Modeling, 21, 329–341. doi:10.1080/10705511.2014.915181
Vermunt, J. K. (2010). Latent class modeling with covariates: Two improved three-step approaches. Political Analysis, 18, 450–469. doi:10.1093/pan/mpq025
References with some innovative uses of LCA
Lanza, S. T., Coffman, D. L., & Xu, S. (2013). Causal Inference in Latent Class Analysis. Structural Equation Modeling, 20(3), 361–383. https://doi.org/10.1080/10705511.2013.797816
Miettunen, J., Nordstrom, T., Kaakinen, M., & Ahmed, A. O. (2016). Latent variable mixture modeling in psychiatric research - a review and application. Psychological MedicinE, 46(3), 457–467. https://doi.org/10.1017/S0033291715002305
Nussbeck, F. W., & Eid, M. (2015). Multimethod latent class analysis. Frontiers In Psychology, 6. https://doi.org/10.3389/fpsyg.2015.01332
References concerning multi-level LCA
Flunger, B., Trautwein, U., Nagengast, B., Luedtke, O., Niggli, A., & Schnyder, I. (2021). Using Multilevel Mixture Models in Educational Research: An Illustration With Homework Research. Journal of Experimental Education, 89(1), 209–236. https://doi.org/10.1080/00220973.2019.1652137
Henry, K. L., & Muthen, B. (2010). Multilevel Latent Class Analysis: An Application of Adolescent Smoking Typologies With Individual and Contextual Predictors. Structural Equation Modeling, 17(2), 193–215. https://doi.org/10.1080/10705511003659342
About the author
Dr Oliver Perra is a lecturer at the School of Nursing and Midwifery, Queen’s University Belfast. His research revolves around the early experiences that explain differences in children’s adaptation and socio-cognitive abilities. He explores these issues by applying a transactional approach: this allows to investigate how interactions between children's characteristics and modifiable environmental factors can affect children's developmental pathways.
- Published on: 22 May 2023
- Event hosted by: Queen's University Belfast
- Keywords: Latent Class Analysis | Mplus | covariates | distal |
- To cite this resource:
Oliver Perra. (2023). Introduction to Latent Class Analysis. National Centre for Research Methods online learning resource. Available at https://www.ncrm.ac.uk/resources/online/all/?id=20806 [accessed: 30 April 2025]
⌃BACK TO TOP