Introduction to Bayesian Analysis
Presenter(s): Andrei Zhirnov
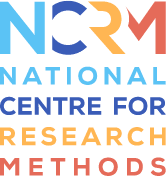
This workshop offers an introduction to Bayesian analysis in R. We will talk about the theoretical underpinnings of Bayesian analysis and the practical considerations for conducting such analyses in R. It looks at some of the methods for specifying Bayesian models, performing the estimation of their parameters and the uncertainty about them, and discuss the ways to interpret and present the results of such analyses using R.
Introduction to Bayesian data analysis
This video introduces this series of videos looking at Bayesian data analysis. Starting with a brief definition of Bayesian data analysis before looking at the likelihood function and the Bayes rule. It provides an opportunity to carry out some manual calculations and doing some simple Bayesian data analysis in R.
Bayesian analysis in a nutshell
In this video, Andrei introduces bayesian data analysis, a way of statistical modelling, looking at what we mean by unknowns and what it means to treat the unknown as random variables.
DGP and Likelihood
In this video, Andrei looks at two concepts that are critical for understanding how we can connect data with the model and the model parameters. Data generating process, which is a model that describes how our data could have been generated. and likelihood function, the probability that we could observe the data set that we actually observed, given the variance of the parameters.
Bayes rule
In this video Andrei walks through an example of data generation using a simple scenario to formulate a likelihood function, specify the prior beliefs, and update those beliefs using the likelihood function constructed.
Bayes rule continued
In this video, Andrei continues to look at the Bayes rule, adjusting the priors to be more appropriate if the analysis was taking place for a different time in the year looking at how the results might differ based on changes to the data available
computations in R
In this final video, Andrei looks at computational approaches to computational methods and how the Markov chain Monte Carlo approach can be used, including an exercise outlining six steps to estimate a logistic regression.
About the author
Andrei Zhirnov is a quantitative political scientist working in the field of Comparative Politics, specifically the comparative studies of political institutions and comparative studies of electoral systems.
Areas of study include:
• institutional and non-institutional determinants of electoral behaviour
• electoral coordination
• organisational stability of political parties
• party system institutionalisation
• interplay between formal and informal institutions
• integrating role of parties in federations
- Published on: 1 July 2021
- Event hosted by: University of Exeter
- Keywords: Quantitative Data Handling and Data Analysis | Statistical Theory and Methods of Inference | Bayesian methods |
- To cite this resource:
Andrei Zhirnov. (2021). Introduction to Bayesian Analysis. National Centre for Research Methods online learning resource. Available at https://www.ncrm.ac.uk/resources/online/all/?main&id=20765 [accessed: 30 June 2025]
⌃BACK TO TOP