Interpreting tables reporting tests of association and tests of effectiveness
Presenter(s): Chiara Dall’Ora
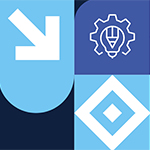
This tutorial explains how to interpret tables that report tests of association and effectiveness in research studies, focusing on understanding statistical results from observational and experimental designs. We will cover how to read and analyse different types of regression models, odds ratios, and effect sizes, distinguishing between statistical and clinical significance.
Associations between variables do not imply a cause-and-effect relationship. There are strict epidemiological rules to establish causality. Experimental studies are usually best placed to meet these rules, although robust observational studies can also demonstrate causality. Generally speaking, an observational study will measure associations, while an experimental study aims to demonstrate a cause-and-effect relationship between an intervention or treatment and an outcome.
Tables that report associations
In an observational study, researchers typically aim to measure the association between one or more variables and an outcome. For more information on the analytical steps used in these studies, there are excellent tutorials on the NCRM website. For example, binary logistic regression is used when the outcome variable is binary, ordinal logistic regression is used when the outcome variable has at least three ordered categories, multinomial logistic regression is used when the outcome variable has at least three categories, and Poisson regression is used when the outcome variable is count data. All regression models share a common feature: they produce an estimate to quantify the magnitude of the association between a predictor and an outcome, allowing us to predict the value of the outcome for any given value of the predictor.
When reading a table reporting associations, follow these steps:
Step 1: Locate the relevant table in the paper. There are often multiple tables reporting tests of association, so make sure to read the labels to identify the correct one.
Step 2: Locate the point estimate for each association. The specific point estimate might vary across papers; it could be a B or beta coefficient, an odds ratio, a hazard ratio, or a rate ratio. The raw coefficient (B) provides information about the effect of each predictor in its original units, while the standardized coefficient (β) allows for comparison across predictors by putting them on a common scale.
Here is an example table showing B and β coefficients:
Table X. Associations between personality traits and willingness to use AI.
B | SE | β | p | |
Neuroticism | 0.27 | 0.05 | 0.21 | .001 |
Extroversion | -0.61 | 0.13 | -0.52 | .001 |
Openness to experience | -0.03 | 0.00 | -0.09 | .001 |
Agreeableness | 0.25 | 0.06 | 0.10 | .001 |
The “B” coefficient value represents the difference in the predicted value of the outcome variable for each one-unit change in the predictor variable. A positive B coefficient indicates a positive relationship between the predictor and the outcome: as the predictor increases, the outcome also increases. Conversely, a negative B coefficient indicates a negative relationship: as the predictor increases, the outcome decreases.
Let us assume that researchers measured the personality trait variables and the outcome variable using validated scales. For neuroticism, this would mean that for every one-unit increase in the neuroticism score, the score on the “willingness to use AI” scale increases by 0.27 units. For extroversion, for every one-unit increase in the extroversion score, the “willingness to use AI” scale decreases by 0.61 units.
The “SE” (Standard Error) of the B coefficient describes the spread of the points from the regression line and measures the accuracy of the estimated B coefficient. It indicates how much the estimated B coefficient is expected to vary due to random sampling variability. The standardized β ranges from -1 to 1 and is useful for comparing all variables as they are now on the same scale. The p-values (the frequency probability of the observed data assuming the null hypothesis) in the table are all <.05, indicating that these associations are statistically significant.
When considering tables that report odds ratios, rate ratios or hazard ratios instead, the overarching principles for interpretation are very similar, but let us consider the table below.
Table Y. Associations between parents’ socio-economic class and students' school enjoyment (yes vs no).
OR | 95% CI
| p | |
Higher managerial (reference category) |
|
|
|
Intermediate | 1.29 | 1.23-1.35 | .0001 |
Lower managerial | 0.97 | 0.94-0.99 | .0001 |
Lower supervisory and technical | 0.98 | 0.09-1.23 | .34 |
OR means odds ratio – it compares the relative odds of the occurrence of the outcome, given exposure to a variable. If the OR values are below 1, the likelihood of the outcome reduces when the predictor is present. If the OR value is exactly 1, it means there is no association. If the value is above 1, the likelihood of the outcome increases when the predictor is present.
In our example, having parents who are in intermediate jobs is associated with a 29% increase in the likelihood of pupils enjoying school, compared to students with parents working in higher managerial jobs (the reference category).
95% confidence intervals of the odds ratios are also important. A confidence interval estimates the precision of the Odds Ratio. It reports the interval where the true odds ratio might be with a certain probability. A large CI indicates a low level of precision of the OR, whereas a small CI indicates a higher precision of the OR. While it is difficult to give a definition of a wide and narrow confidence interval, if the values of the confidence interval overlap 1, the association between the predictor and outcome is not statistically significant. In our example table, the association between having parents that work in lower supervisory and technical jobs and students’ school enjoyment is not statistically significant.
A note on Odds Ratios and other measures of relative risk: the raw counts are important when interpreting odds ratios. For example, a 29% increased likelihood of students enjoying school if their parents work in intermediate jobs could sound like a large association, but it is important to know the absolute numbers. In our example, 9 out of 125 (7.2%) students with parents in the intermediate jobs enjoyed school, while 7 out of 123 (5.6%) enjoyed school in the higher managerial job. This figure with absolute risk sounds less striking than “a 29% increase” – the relative risk. This is a useful reminder of why descriptive data (see video 1 of this resource) are important.
Step 3: identify whether the results reported are unadjusted or fully adjusted – unadjusted models report associations between one variable only and the outcome, while fully adjusted models report associations between one or more variables and the outcome while controlling for (or adjusting for) a number of other variables that have been deemed as potentially influencing the association between a predictor and the outcome. In a high-quality paper, you will find both unadjusted and adjusted models. Here is an example table illustrating how this might look:
Table W. Associations between parents' job status and students' school enjoyment.
Univariable associations | Full model* | |||||
OR | 95% CI
| p | OR | 95% CI
| p | |
Higher managerial (reference category) |
|
|
|
|
|
|
Intermediate | 1.29 | 1.23-1.35 | .0001 | 1.11 | 1.06-1.28 | .001 |
Lower managerial | 0.67 | 0.62-0.80 | .0001 | 1.01 | 1.01– 1.05 | .001 |
Lower supervisory and technical | 0.98 | 0.95-1.23 | .34 | 0.93 | 0.89–1.12 | .20 |
* Adjusted for age, gender, quality of peer relations on a scale ranging from 1 “poor” to 4 “excellent”) |
The point estimates change when the analysis controls for other variables (these are specified at the bottom of the table, but sometimes they are described in the manuscript within the statistical analysis section). In one case, for students with parents in lower managerial jobs, the ORs change direction. This indicates that when taking into account students’ age, gender, and quality of peer relationships, students with parents in lower managerial jobs are more likely to enjoy school than students with parents in higher managerial jobs.
Tables that report measures of effectiveness
The main analysis principles that guide reporting of experimental research results are broadly the same of observational research, but the tables reporting these results are slightly different. In these tables, you will see columns comparing results of the intervention / experimental group to those of the control group(s).
Step 1: Locate the table with the results you are looking for. Some studies report more than one outcome, so ensure you are reading the correct table. Sometimes, if researchers have collected outcome data at more than one time point post intervention, you might find figures to aid visualisation of the results.
Step 2: Recall the data analysis plan to identify what type of effect size you might expect to see. For example, in studies that measure outcomes as numeric variables, you might expect to see the means and standard deviations reported, while with dichotomous outcomes you would look for odds ratios. You should find a point estimate (and confidence interval when odds ratios are displayed) for each time point the outcome was measured at post intervention.
Step 3: Consider the magnitude of the effect the intervention has had on the outcome. See Table Z below for an example.
Table Z. Quality of life per time point by group and overall, mean (SD).
Mean (SD) |
| ||||||
Intervention group (life coaching post cancer diagnosis) | Control group (standard care) |
| |||||
T0 n=772 | T1 n=768 | T2 n=749 | T0 n=791 | T1 n=785 | T2 n=771 | p | |
Quality of life* |
|
|
|
|
|
|
|
Physical | 62.6 (11.1) | 63.9 (9.8) | 64.2 (9.1) | 63.1 (9.9) | 63.7 (8.4) | 64.1 (8.5) | .01 |
Psychological | 59.1 (12.3) | 60.1 (11.4) | 60.2 (12.8) | 59.3 (6.8) | 60.0 (8.7) | 60.1 (9.1) | .01 |
Social relations | 63.6 (15.8) | 64.9 (10.9) | 67.7 (14.3) | 63.5 (14.7) | 64.9 (9.2) | 67.8 (8.9) | .21 |
* Adjusted for age, gender, ethnicity, socioeconomic status, job status (unemployed/employed/retired/semi-retired) |
In the intervention group, the mean physical dimension of quality of life has increased from 62.6 to 64.2 at time-point 2 post-intervention. For the control group, there has also been an increase in physical quality of life, from 63.1 at T0 to 64.1 at T2. Remember that these are not crude estimates, meaning, the researchers have not just measured the mean and standard deviations at different time points and reported them in the table. The point estimates you see in the table derive from a linear mixed model where researchers adjusted for a number of demographics that might influence quality of life beyond the effectiveness of the intervention. We can see from the p-value .01 that the effect of the intervention was statistically significant overall. If we consider social relations, the p value .21 indicates that we cannot reject the null hypothesis, there are no statistically significant differences in outcomes between groups. You might find that researchers report different point-estimates, for example, marginal means and confidence intervals instead of standard deviations.
As a final remark, go beyond statistical significance when reading about the effectiveness of a treatment. Consider the clinical significance of the results, for example, is a decrease of 1.5 points on a scale enough to upscale and implement the intervention? On a scale ranging from 1 to 100 it might be a negligible difference, while on a scale ranging from 1 to 10, it might be an important decrease. When reading results from a trial, consider whether researchers have conducted an intention-to-treat or per protocol analysis. Intention to treat refers to including all the participants randomised in the trial, regardless of whether they completed the trial in the analysis, while “per protocol” refers to including only participants who have completed the trial.6 Intention-to-treat is generally preferred because it removes the consequences of participant crossover and dropout, which may break the assumption of random assignment to the intervention/experimental and control groups in a study.
About the author
Chiara qualified as a Registered Nurse in 2011. In 2018, she was awarded a PhD in Health Sciences at the University of Southampton. Since 2023, Chiara has been an Associate Professor within the Health Workforce & Systems theme in the School of Health Sciences. Chiara leads a research programme to improve health workforce wellbeing and performance, with a specific focus on work hours and workforce configuration and patient safety. She draws on large workforce and patient datasets and applies multilevel modelling techniques.
- Published on: 20 November 2024
- Event hosted by: Southampton
- Keywords: Descriptive statistics | inferential statistics | significance testing | Descriptive Statistics | Statistical Theory and Methods of Inference | Experimental Research | Associations | regression | causality |
- To cite this resource:
Chiara Dall’Ora. (2024). Interpreting tables reporting tests of association and tests of effectiveness. National Centre for Research Methods online learning resource. Available at https://www.ncrm.ac.uk/resources/online/all/?id=20847 [accessed: 18 July 2025]
⌃BACK TO TOP