RADIANCE - Machine Learning and Causal Effects
Date:
10/06/2024 - 12/06/2024
Organised by:
University College London
Presenter:
Dr Eduardo Fe
Level:
Intermediate (some prior knowledge)
Contact:
Olivia Evans - olivia.evans@ucl.ac.uk
Dr Eduardo Fe - eduardo.fe@manchester.ac.uk
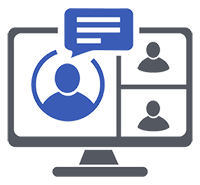
Venue: Online
Description:
Course description
This intermediate course offers a comprehensive understanding of the links between machine learning and traditional methods in causal inference. This course was designed for participants that are familiar with causal inference and the fundamentals of Machine Learning.
Key topics covered:
Potential Outcomes: Understand the concept of potential outcomes and how machine learning can be applied to estimate causal effects in observational studies.
Matching and Instrumental Variables: Explore advanced methods such as matching and instrumental variables to address confounding variables and strengthen causal inference models.
Trees and Regularisation: Learn how decision trees and regularisation techniques can be integrated into causal analysis, providing participants with tools to handle complex datasets and improve model performance.
Learning objectives
There are two main learning objectives:
To develop an understanding of how Machine Learning is being applied for causal inference in real-world studies.
To be able to implement some of the available methods in R.
Course Structure
There are three scheduled live online sessions, each consisting of a lecture and a hands-on computer practical session. During the practicals, participants will be provided with materials to implement the methods discussed in the respective lecture. Solutions will also be given to enable them to easily apply what they learn to their own work.
Timetable
Date | Time | |
Monday 10th June 2024 | 9:30am-12:30pm | Live session and practical 1 |
Tuesday 11th June 2024 | 9:30am-12:30pm | Live session and practical 2 |
Wednesday 12th June 2024 | 9:30am-12:30pm | Live session and practical 3 |
Pre-requisite
Participants should be familiar with causal inference, including key concepts such as confounding, treatment effects, and model evaluation is essential. In addition, attendees should have basic knowledge of Machine Learning to the level of the following suggested reading list:
James, G., D. Witten, T. Hastie, R. Tibshirani (2021) Introduction to Statistical Learning with Applications in R, 2nd Edition. Springer Texts in Statistics. Available for download at: https://www.statlearning.com/
Neal, B (2020) Introduction to Causal Inference from a Machine Learning Perspective (Lecture notes). https://www.bradyneal.com/Introduction_to_Causal_Inference-Dec17_2020-Neal.pdf
Hernán MA, Robins JM (2020). Causal Inference: What If. Boca Raton: Chapman & Hall/CRC. Available for download at: https://www.hsph.harvard.edu/miguel-hernan/causal-inference-book/
Cost:
£90 per person and £45 for PhD students
Website and registration:
Region:
International
Keywords:
Quantitative Data Handling and Data Analysis, Data Mining, Machine learning
Related publications and presentations from our eprints archive:
Quantitative Data Handling and Data Analysis
Data Mining
Machine learning