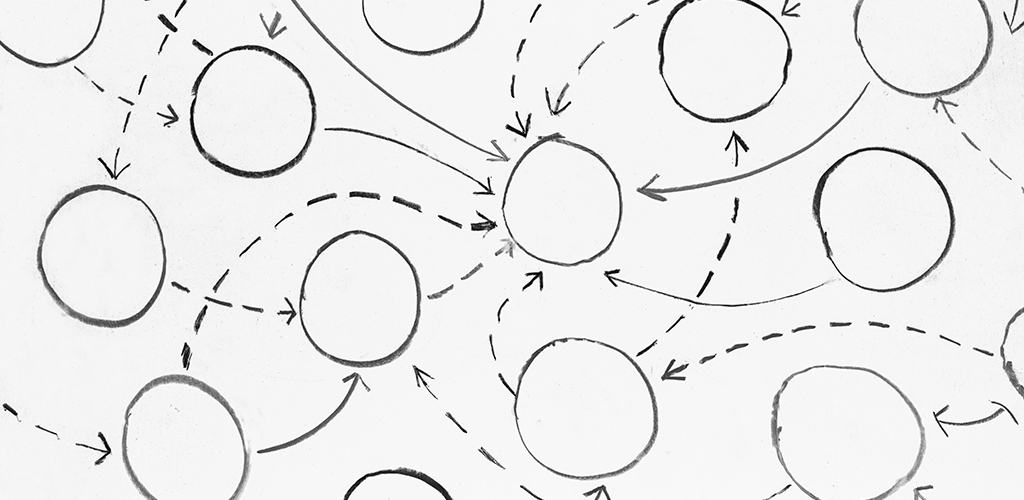
Most of the time, social science researchers analysing quantitative data will tend to fit statistical models which explore variation in a single outcome variable. For example, in my own work I look at educational outcomes, such as the number of qualifications a young person has attained or whether they attended university or not. Using models in the generalised linear modelling framework, such as linear regression or logistic regression models, helps us to understand variation in these outcomes, accounting for multiple explanatory variables that are associated with the outcome. For instance, we might be interested in knowing whether ethnic group differences in educational attainment persist once we have controlled for social class.
The outcome variables we analyse are measures (or indicators) of underlying concepts. For example, an IQ test is a standardised test designed to measure the concept of intelligence. We cannot directly observe intelligence, so we have to use a measure which we think maps onto the concept. But what if we have several measures in our data that we believe relate to our concept? Are these indicators measuring the same thing? How consistent are the responses that people give to questions on a similar topic?
Latent variable techniques attempt to answer these questions. A latent variable is one that cannot be directly observed but is estimated based on a series of observed variables (for example, perhaps using a battery of survey questions on a similar topic). Latent variable models can help us to understand the patterns of association between sets of variables. Another way of understanding these techniques is that latent variable models seek to explain the relationship between multiple correlated observed variables using a common underlying latent variable
There will often be a high number of possible response patterns that people give to survey questions. Particular latent variable techniques allow us to examine whether there are groupings within the responses provided. Other latent variable techniques instead consider the latent variable as a continuous scale. Alternatively, the responses given to a set of survey questions might be recorded on a scale rather than a set of categories. How do we handle this?
The short answer is that the type of statistical model we fit depends on how our variables are measured. This is true of latent variable models. Different models have been developed to account for how we understand either the latent variable or the observed variables to be expressed. Once we have an elementary understanding of one type of latent variable model, we can start to understand how to estimate and interpret other types of model.
Learn more about latent variables
If you are an empirical social science researcher with knowledge of statistical data analysis methods but want to learn more about latent variable models, then the following online one-day course (on 14 September 2022) will provide an overview of the different latent variable techniques and how they are related. The demonstration and practice exercises will use Stata. Familiarity with Stata is helpful but not essential for participants on this course.
What will be covered?
The course, Latent Variable Models for Social Research, covers:
- Introduction to latent variable models
- Comparison of factor analysis, latent trait analysis and latent class analysis
- Estimation and interpretation of latent class models using Stata
- Practical exercise: Estimation of latent variable models in Stata
What can attendees expect to gain?
By the end of the course participants will:
- Gain a clearer understanding of the concept of latent variable models
- Be able to select an appropriate latent variable model
- Estimate and interpret latent class models using Stata
- Be aware of potential issues during model estimation
Meet the presenter
Dr Chris Playford is quantitative sociologist at the University of Exeter working in the fields of social stratification and the sociology of education. His work has focused on modelling the role of family background on educational attainment with a substantive interest in inequality and disadvantage. He specialises in the secondary analysis of large-scale survey and administrative data.
Dr Playford has methodological interests in a range of statistical techniques including generalised linear and mixed models, latent class analysis and multiple imputation of missing data. In a previous role he researched child development and emotional well-being. He has also published work on research reproducibility.
Register for the course, Latent Variable Models for Social Research