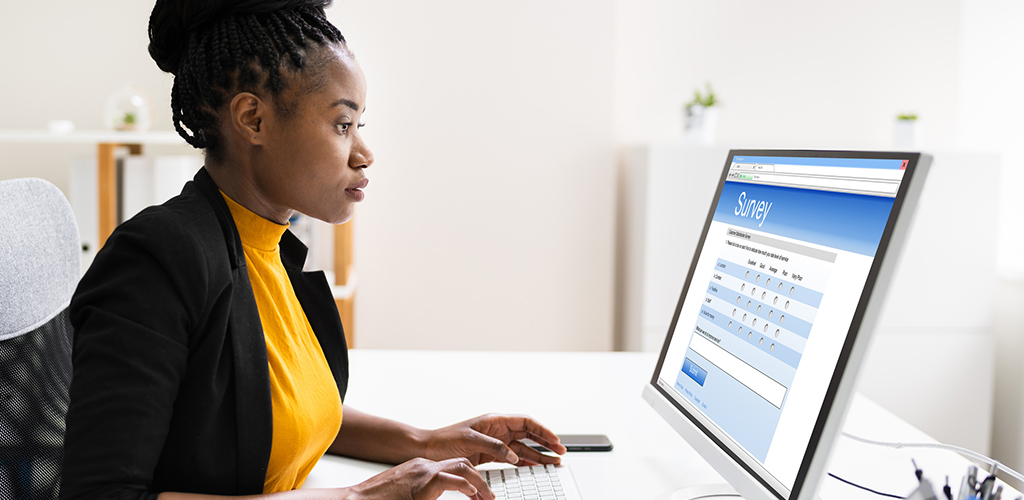
Since the beginning of the COVID-19 pandemic, we have learned a lot about how the global spread of the virus has impacted people’s lives. Surveys have played a particularly large role in gathering this knowledge. Among other aspects, surveys have asked people about how the pandemic affects their mental health, how they organise child care when schools and kindergartens are closed, and to what extent they support and adhere to social distancing rules. However, it is difficult to assess which data to trust among the myriad of available data sources and their potentially incoherent findings.
For many decades, it was universally accepted to trust survey data if the survey participants were randomly selected from the general population – the so-called probability sample survey. The statistical theory explaining why and how valid inferences can be drawn from probability sample survey data is well-established and a universally accepted framework for assessing and correcting potential errors exists. However, since the beginning of the 21st century, the trust in probability sample surveys has been put into question by two major developments: (1) the decline of probability sample survey response rates across the globe, and (2) the availability of fast and cheap nonprobability survey data collected on the internet.
Declining response rates challenge probability sample surveys, because of the potentially non-random nature of the survey nonresponse. Empirical evidence suggests that people who do not comply with survey participation requests may differ from people who do comply. If the nonresponse bias cannot sufficiently be corrected, inferences drawn from the data may well be inaccurate. Additionally, the availability of fast and cheap nonprobability online survey data challenges traditional probability sample surveys, because in some situations it can be argued that imperfect data beats no data at all. For example, at the onset of the COVID-19 pandemic, many probability sample survey programmes were too slow or collected data too infrequently to fill the urgent data demand.
Nonprobability surveys were much faster in providing pandemic-related data. This is because nonprobability surveys usually rely on self-selected volunteers recruited on the internet. Oftentimes, this recruitment works via advertisements placed on social media timelines, in pop-up windows and banners which appear on a vast variety of websites, or may even be embedded as interactive features in news articles. Internet users who click on the ad either participate in a one-off survey or, more frequently, are asked to register to an online panel. Nonprobability online panels are particularly efficient in collecting fast and cheap data, because they recruit large pools of online volunteers, who they can invite to multiple surveys as needed.
While conveniently available, nonprobability online surveys have repeatedly been shown to produce less accurate results than probability sample surveys. Furthermore, because inference from nonprobability survey data commonly relies on untestable assumptions, it is questionable whether important societal decisions, for example on political interventions during the pandemic, should be based on them.
Nevertheless, the last years have seen a number of notable advancements in nonprobability survey methodology. Super-population modeling, fit-for-purpose designs, and blended calibration techniques are among the keywords which are relatively new to the debate. Furthermore, nonprobability surveys fill an important gap in surveying hard-to-reach and special populations, including ethnic and sexual minorities, using techniques such as Respondent Driven Sampling and targeted survey advertisement. My course, (Non-)Probability Survey Samples in Scientific Practice, is designed to help applied researchers and survey practitioners to make evidence-based decisions on designing, using, and evaluating (non-)probability sample survey data.