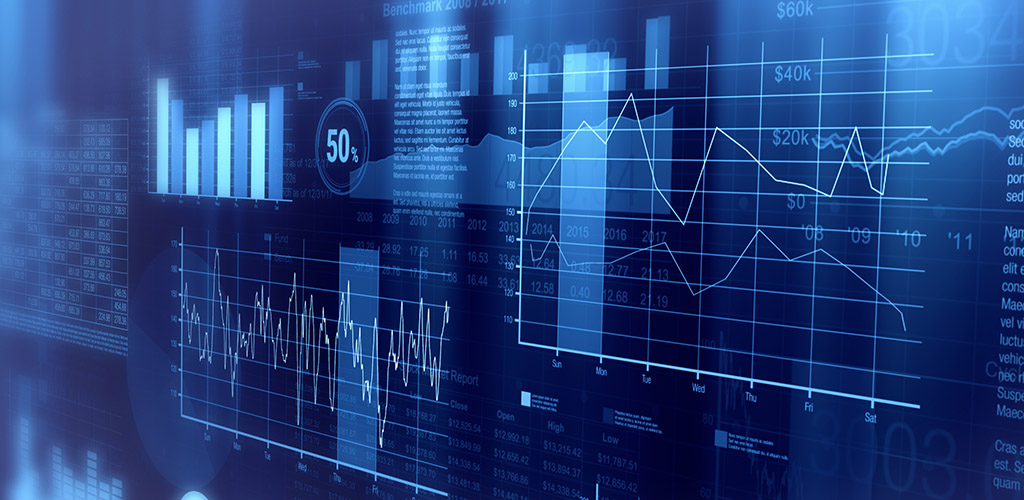
In many practical situations when it comes to modelling data through a simple linear regression, we may have the scenario where observations are somehow similar, correlated or grouped according to some features. Then, the independency assumption is violated and the linear regression will no longer be reliable and perhaps not a good choice. There are many occasions where this could happen in practice; however, ignoring this relatedness at the analysis and interpretation stage is problematic and leads to unreliable results.
Take for example the maths marks of pupils in a school. The marks of pupils who have been taught by the same tutor will be grouped according to some features. The immediate question is, if we apply a simple linear regression, then how do we assess and examine this similarity, its magnitude and role in explaining the variation in maths marks within and between tutors? This is why we need the concept of multilevel models (MLM), also known as mixed-effects models.
We are a group of statisticians and quantitative methods researchers at the Durham Research Methods Centre (DRMC) at Durham University who have experience working on clinical and educational trials. We have seen that most of the time in analyses of these trials, observations follow a kind of hierarchal data structure or, in other words, they might be grouped or clustered according to some common feature(s). The knowledge of how to deal with such data would be a non-negotiable essential skill that researchers in this area must have.
We are excited to propose a special course on the concepts and applications of MLM based on what we have observed and learned over the course of our research. To complement what can be well found in lots of books on MLM, we will focus on how we deal with this concept in practice and how we apply it when working with real data. The course will be held online on 25-28 October 2021.
Transitioning from linear regression models to multilevel models
This course’s focus will be on the conceptual understanding of multilevel modelling and its relevance for robust analysis of evidence from cluster randomised trials (CRT) and longitudinal data structures. It will focus on ‘meaning’ and application of multilevel models instead of computations, using lectures, small group breakout sessions and practical sessions.
The course will run for four days with the first day focusing on the transition from linear regression models to multilevel models. Practical examples with simple exercises will be used to motivate the need for a more robust approach than t-tests or linear regressions in CRTs. The different sources of variability will be discussed as well as their implications on effect size. The course will primarily be taught in R.
This is an intermediate course that requires good understanding of the linear regression model as a prerequisite. This course is suitable for postgraduate students and researchers interested in the analysis of cluster randomised controlled trials and hierarchical/longitudinal data with a basic understanding of statistical methods including analysis of variance and the linear regression model.
What will be covered?
The course covers:
- Overview of multisite and cluster randomised controlled trials
- Hierarchical or correlated data structures including longitudinal data
- Linear regression with structured covariances
- Random intercept models
- Random site by intervention models.
What can attendees expect to gain?
By the end of the course participants will:
- Gain practical skills in converting data to long form
- Make links between study design and analytical choice
- Gain practical skills in applying multilevel models and interpreting results
- Acquire necessary skills to check robustness of results.
Meet the presenters
Professor Jochen Einbeck (Co-Director of DRMC): I completed my PhD in Statistics in the year 2003 at the University of Munich. This was followed by an SFI-funded position as a postdoctoral researcher at the National University of Ireland, Galway, where I implemented the mixed effect package R npmlreg which is available on CRAN. Since 2006, I have been based at Durham University, initially as Lecturer and since 2020 as Professor of Statistics. I have developed a number of postgraduate and short courses, and delivered these at multiple occasions, such as at the CMStatistics 2019 conference, as well as at multiple universities in Brazil.
Dr Bilal Ashraf (Fellow of DRMC): I am a postdoctoral research associate in statistics. Astatistical geneticist by training, my key expertise is in genomic prediction and Genome Wide Association Studies (GWAS) of human and non-human species. I have contributed to various research projects in plant, animal and human genetics. I have experience of analysing large-scale datasets in health and social sciences. I am currently exploring the application and development of statistical methods in applied and social and health sciences. My current work is on a project funded by the Education Endowment Foundation- that is exploring individual participant data meta-analysis of the impact of educational interventions on certain group of pupils.
Dr Akansha Singh (Fellow of DRMC): I completed my Master’s degree in Statistics and obtained my PhD in Population Studies from International Institute for Population Sciences, India. I worked as a post-doctorate researcher in Catholic University of Louvain, Belgium and have been working as a post-doctorate research statistician in Durham University since 2018 on a project with the Education Endowment Foundation focused on developing methods and analysis of education trials. My area of research interest is the development and application of advanced statistical and data science methods to address research questions in the area of education and health.
Dr Janelle Wagnild (Fellow of DRMC): I completed my MSc in Evolutionary Medicine (2015) and PhD in Biological Anthropology (2019) at Durham University. During my doctoral training, I became interested in the applications of various advanced quantitative methods to address key research questions in the anthropology of health. Since 2019, I have been in post as a Postdoctoral Research Associate in Quantitative Methods at Durham in which I do statistical analyses of data gathered from clinical and non-clinical contexts and work to develop new applications of advanced quantitative methods for health research.
Dr Ehsan Kharati (Fellow of DRMC): I graduated with a Master’s degree in Mathematical Statistics from the Department of Statistics at Shiraz University, Iran and completed my PhD in Statistical Sciences from Padova University, Italy, focusing on applied statistics in signal and brain image analysis in public health. I joined Durham University in 2018 as a Research Associate in Biostatistics. My main research interests are the development of advance statistical methods in design and analysis of (cluster) randomised controlled trials in public health and social care.
The delivery of the course will also be supported by Dr Nasima Akhter, Assistant Professor in quantitative methods in the Department of Anthropology and Dimitrios Vallis, Research Assistant and research fellow of DRMC.