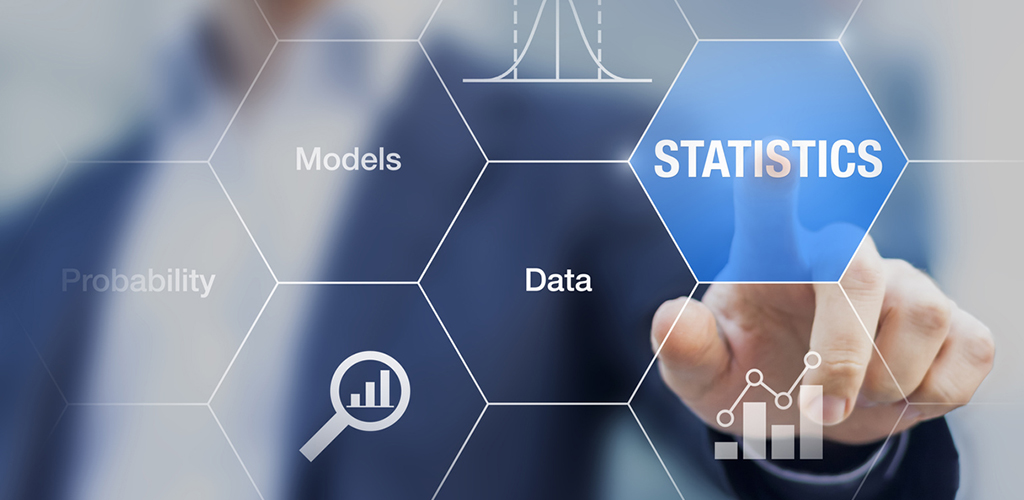
This week I am just back from teaching a two-week course at the Essex Summer School called Introduction to Multilevel Models. Traditionally a popular annual residential summer school that mostly attracts doctoral and early-career post-doc students from across Europe and beyond, in 2020 and 2021 the ESS had been held through online course delivery – a format with pros and cons but which more than filled up all of my free time during the two weeks that my own course was running.
Personally I’m now into my 11th year of teaching on multilevel models at Essex, but I’m always heartened to see the range of pedagogic needs that courses at the Essex Summer School usually manage to support. By offering relatively long courses in moderately advanced topics in social science methods, undertaken in a relaxed, inter-disciplinary environment, courses are, in my view, typically able to provide their students with skills and insights that span traditional dualities of depth and breadth; theory and practice; critique and operationalisation.
Addressing the full spectrum of challenges
I’ll be doing my best to hit a similar sweet spot in a forthcoming two-day online course, Random Effects Modelling – Advanced Issues, that I’m preparing to run within the NCRM short course programme. It runs on 18-19 August 2021.
Drawing upon my previous experiences of teaching on multilevel modelling, I’m planning to cover a number of challenges and enduring debates that impact applied research that uses random effects models. In spite of the course title, it is not my intention to cover really extended issues of statistical model specification; by contrast my interpretation of “advanced” is more often about dealing with the full spectrum of challenges associated with using random effects models productively in an applied research project.
To me, that does require coherent conceptualisation, but placed right beside a range of important practical considerations such as awareness of data and software opportunities, and the implications associated with making different choices of model specification or relevant data assumptions. The course accordingly will have both lecture and illustrative online lab sessions, and most of its sessions will be directed in some way to the task of making and justifying difficult operational decisions about the best way to model the empirical patterns within a dataset.
Illustrating practical issues
In case you’re not used to the terminology, the focus on random effects models – aka mixed models – concerns the particular device of specifying model parameters that describe systematic patterns within a model’s error terms. When most people in social statistics refer to multilevel models or hierarchical models, they usually mean random effects models, although there is an argument that this doesn’t follow automatically, as there are other strategies for modelling multilevel or hierarchical structures that do not involve the use of random effects.
In the course that I’m planning for 18-19 August, lectures will cover terms and concepts, different traditions of use in different social science disciplines, debates on whether or not to use random effects terms including alternative fixed effects models and alternative estimation strategies. I’ll also be covering scenarios when we work with complex data structures that may feature multiple complicating features. Some lab materials will also be prepared that are designed to illustrate practical issues and offer training in implementing random effects models appropriately.
Analysing complex situations
The ambition is to cater, like the Essex Summer School does, to the substantial midfield of applied social researchers using quantitative datasets – that is, those of us who have a fair amount of previous experience in statistical analytical methods and an interest in analysing quite complex situations, yet are social scientists rather than statisticians, and value insights into getting applied work done in complex settings. If that sounds like it could be you, your colleague, or your doctoral student, consider coming to, or sending them along to this one!